Discrete Choice Model
Discrete Choice Model Surveys can Simplify Complex Product Feature Decisions
What is a Discrete Choice Model?
Discrete Choice Modeling or Discrete Choice Experiment is a research method and statistical technique used by researchers and marketers worldwide.
Often referred to as Conjoint Analysis, it is a way to examine any choice situation being made by decision-makers based on various product characteristics or available choice set features, pricing, and brands.
When planning your market analysis roadmap it is ideal for new product development, advertising/promotion, and pricing.
Marketers and product managers can use discrete choice models to effectively optimize their offerings and gain distinct competitive advantages over their competition.
Two key deliverables:
- Share of preference or an estimation of the choice probability of user’s ordered choice picks
- A utility function for each feature/variable ranked from a choice set
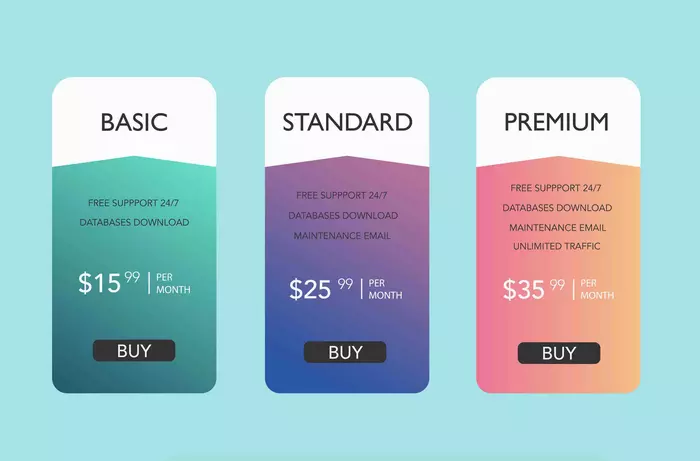
What are the common use cases for a discrete choice model?
Discrete choice models are widely used across many industries today, including consumer goods and services and B2B applications.
You can find mobile phone manufacturers using these techniques to optimize offerings based on characteristics like screen resolution, phone size, and camera quality.
Banks create discrete choice models to determine the optimum bundle of accounts and services to attract new customers.
At OvationMR, we offer a special focus in the area of B2B research. We’ve helped many B2B commerce companies make decisions with discrete choice modeling across various product categories.
Here are 4 examples where we made an impact
A large industrial fan company to optimize a new series of ceiling fans for manufacturing and warehouse spaces. Product configurations were optimized for segments of users based on their characteristics:
- Type of Building
- Size of Business
- Usage of Other Air Movement Products.
A B2B equipment company where a discrete choice analysis determined the optimum array of standard product color offerings for maximum sales potential within a specified cost containment target. The product was successfully launched.
A first responder equipment company to identify the ideal set of product features within a targeted price range for a search and rescue-related device, still in development.
A commercial HVAC company to help them create a comprehensive climate control system still in development with the help of a series of discrete choice studies.
The discrete choice modeling concept
Nearly all purchase decisions include choices based on several factors. Suppose you are in the grocery store and want to buy a bag of coffee. You pick up two bags to compare.
- Brand A is from Nicaragua, is a dark roast, whole bean, and costs $8.99.
- Brand B is from Ethiopia, mild with hints of hickory, is pre-ground, and costs $9.49.
- Additionally, the brand donates 5% of its profits to help supply villagers with drinking water.
You weigh these various attributes as you decide on your purchase. There is a strong probability that certain attributes are more important to you than others.
Every consumer is different, and it is impossible to predict what choices any one person will make. By interviewing enough people, we can identify patterns of decision-making and develop conclusions about what attributes matter to decision-makers.
We also learn just how critical each of those attributes is in the purchase decision process. Each attribute carries a certain level of importance or weight, and indeed certain levels of attributes are more valued than others (i.e., a $100 price might be more important than a $125 price, for example).
Example discrete choice modeling study
A discrete choice modeling study is designed to uncover the values assigned to the various product features. To get there, we must follow these steps:
Identify buyer perceptions and purchasing behaviors – This can be done via a combination of qualitative research, observational research, and secondary data research. Qualitative research might include focus groups or IDIs.
Identify all possible features that might be included in the product or service – This is typically done during brainstorming among the research team and should include product managers, salespeople, and other appropriate staff.
We worked with a client product team to identify over 100 potential product features on a recent project
Reduce the number of possible features to a manageable number – Alternatives are good but can be overwhelming. A typical decision-maker might consider four to eight attributes. Some features might be eliminated upfront due to developmental obstacles (e.g., technology does not exist yet) or cost issues.
Others might be eliminated by conducting market surveys among decision-makers to have them choose the items that are most (and least) important.
Once the feature set is identified, the core research is then conducted – After designing your choice modeling survey, settling upon your target audience and determining your sample size, respondents are presented with a set of choice experiments.
They are told to imagine they are in the market for the item and can choose from a series of options or reject them all.
As the survey progresses, respondents are shown a variety of options with changes made to the levels for each feature. A typical study might involve showing each respondent eight to 12 such choice options.
Here’s an example from a robot vacuum study:
The options presented are by design and ensure that a good representative mix of product configurations is achieved. Over the course of a number of respondents, the researcher can begin to get a sense of how people make tradeoffs between product attributes.
Note the “none” option. This is important! Your prospects may reject all product choices, just as they may in the marketplace.
Discrete choice modeling has advantages over traditional research approaches
A discrete choice modeling approach delivers where traditional methods fall short. Discrete choice models enable the researcher to discern demand for product features among selected consumer segments. From this, an array of products can be developed to fully address market potential.
Discrete choice modeling techniques allow the researcher to test, in quite an efficient manner, feature combinations, and pricing outside of current marketplace offerings. The researcher is not limited to current offerings.
Discrete choice model output
The key deliverable of this type of research is a model that the researcher (and client team) can use to create what-if scenarios and estimate the share of preference among prospective buyers.
The model can also be used to develop product configurations that optimize profitability or revenue.
Based on each product configuration tested, the model calculates shares of preference. Here’s an example from the Robot Vacuum study:
Discrete choice modeling research is an excellent way to simulate pricing effects. In this example, the share of preference is calculated for a specified product configuration at varying prices:
In addition to the product configurations tested, the researcher can “build” new products in the simulator to model what might happen if such a product were introduced to the market.
A utility report is also generated to help the team understand the relative importance of selected product attributes.
Putting the Results to Use
Discrete choice modeling is a seriously powerful tool that can be used in a number of areas:
- Marketing teams can use the tool to determine pricing and discount options.
- Product management can predict outcomes based on competitor actions.
- Marketing departments can develop advertising which emphasizes the features most important to buyers and can tailor these by customer segments.
- Sales departments can develop sales pitches and collateral to sell by feature set.
- Finance can conduct analyses to estimate revenue and investment needed to reach sales goals.
- And more!
Follow
OvationMR
Bart Borkosky
Author
Bart Borkosky is Chief Research Officer of OvationMR and posts frequently on The Standard Ovation and other Industry Blogs.
OvationMR is a global provider of first-party data for those seeking solutions that require information for informed business decisions.
OvationMR is a leader in delivering insights and reliable results across a variety of industry sectors around the globe consistently for market research professionals and management consultants. Visit: https://www.ovationmr.com.
Need help with your project?
We are ready to offer you: